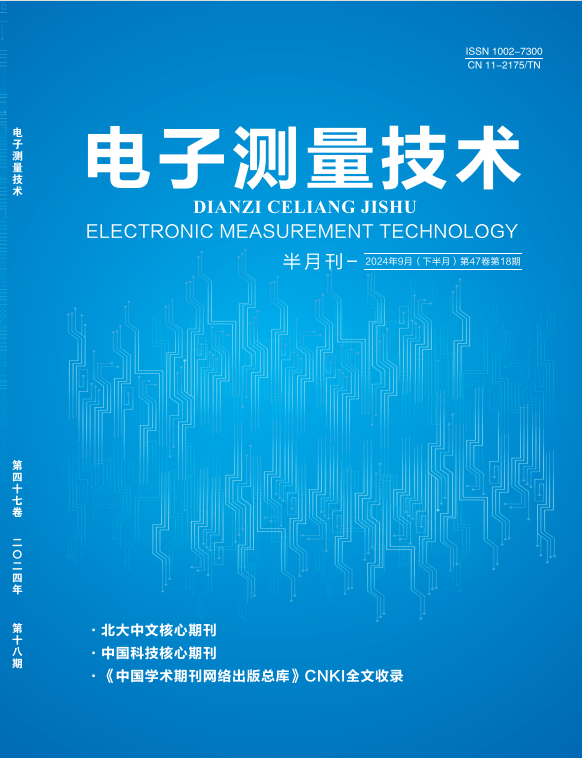
Editor in chief:Prof. Sun Shenghe
Inauguration:1980
ISSN:1002-7300
CN:11-2175/TN
Domestic postal code:2-369
- Most Read
- Most Cited
- Most Downloaded
Li Ran , Xing Liyun , Pang Na , Shen Jianqiang , Wang Ce
2024, 47(10):1-9.
Abstract:In order to solve the problem that the current-based midpoint-clamped three-level inverter open-circuit fault diagnosis is easily affected by the load changes, this paper mainly improves the accuracy from the fault feature differentiation. Firstly, VMD improves the modal aliasing phenomenon, and its optimal modal number K and penalty coefficient α are optimized by SMA, which improves the independence of fault features. Second, due to the relatively smooth distribution of wavelet packet energy, which can effectively overcome the characteristics of load influence, the maximum value of the two-layer wavelet packet energy of each IMF is taken as the fault feature quantity, so that the time-frequency feature information is more centralized which further improves the fault feature differentiation without the influence of varying loads. Finally, the fault features are applied to the neural network for training, and the weights and thresholds of the model are optimized by SSA, which solves the problem of local optimum of the model and improves the accuracy of fault identification. Through the simulation experimental results of 17 open-circuit faults in the NPC three-level inverter circuit model, the diagnostic accuracy of this method reaches 98.99%, which is applicable to the online fault diagnosis of NPC three-level inverters under variable load conditions.
Sui Xiuwu , Fu Shixiong , Liu Jinyu , Wang Tao , Liu Yang
2024, 47(10):10-18.
Abstract:Aiming at the problem of much noise interference in the acquired surface EMG signals and the lack of neck and shoulder muscle fatigue state classification standard and classification model, this paper proposes a neck and shoulder muscle fatigue classification method based on joint denoising and optimized limit learning machine. First, AnyBody was used to establish a biomechanical model of neck and shoulder skeletal muscles, and the muscle fatigue state was classified according to the muscle pH value and the RPE exertion perception scale. Surface EMG signals were collected from six healthy young people in the fatigue state of the upper trapezius muscle bundle. Then, joint denoising was performed by combining Kalman filtering and improved wavelet threshold function to extract six feature parameters: root mean square, integrated electromyography, mean power frequency, median frequency, instantaneous mean power frequency, and instantaneous median frequency. Finally, the weights and thresholds of Extreme Learning Machine were optimized using Improved Whale Optimization Algorithm to establish the IWOA-ELM neck and shoulder muscle fatigue classification model. The experimental results show that the joint denoising algorithm is more effective, and the accuracy of the IWOA-ELM model is 96.3% in the training set and 97.5% in the test set, with a root mean square error of 1.108, and the accuracy of the classification model is higher than 93% for different subjects, so the joint denoising algorithm and the IWOA-ELM model proposed in this paper have an advantage in classifying the fatigue of neck and shoulder muscles.
Zhang Yu , Li Jianxin , Zhu Yongjian , Ma Teng
2024, 47(10):19-26.
Abstract:In recent years, deep learning has achieved breakthrough progress in radar target recognition. However, research on deep learning target recognition algorithms based on radar cross-section (RCS) data is relatively scarce. Additionally, space target radar signals are easily affected by noise, resulting in low target recognition accuracy. This paper proposes an end-to-end Time-Frequency Feature Fusion Neural Network (TFF-Net) for space target recognition based on RCS sequence data. First, time-frequency analysis methods are used to convert the RCS sequence data into two-dimensional time-frequency data to reduce noise interference. Then, TFF-Net is used to extract deep features from the time-frequency data. TFF-Net first uses a convolutional neural network to capture spatial features of the targets, then employs a bidirectional long short-term memory network to model temporal information, and finally applies a temporal attention network to adaptively focus on important sequences in the time-frequency data. Comparative experiments were conducted on a space target dataset. The results show that the proposed algorithm achieves a space target recognition accuracy of 95.8%, significantly higher than several current mainstream radar target recognition algorithms. Furthermore, the classification accuracy under low signal-to-noise ratio conditions is also superior to other algorithms, demonstrating better noise robustness.
2024, 47(10):27-33.
Abstract:Compared with traditional inertial sensors and visual sensors, using millimeter-wave radar for human activity detection not only requires low environmental demands and high sensitivity but also can effectively address privacy leakage issues associated with cameras. To tackle the problems of clutter interference and high complexity of network models in current millimeter-wave radar human activity detection, this paper proposes improvements and quantization processing of existing residual neural network. By integrating radar time-frequency transformation and clutter suppression, a complete signal processing flow for radar human activity detection is presented. The time-frequency transformation section adopts range dimension FFT, high-pass filtering in slow-time dimension, and short-time Fourier transformation to obtain Time-Doppler spectrum. The residual network section embeds the CBAM attention mechanism and quant it from 32 bits to 8 bits. Finally, the Time-Doppler spectrums are input into the network model for feature extraction and classification to obtain detection results. Experimental results demonstrate that this method can eliminate interference from static clutter, achieving a detection accuracy of 97.33% with a model size of 20.2 MB.
2024, 47(10):34-47.
Abstract:Oblique photography technique is of great significance for generating large-scale scenes. In order to address the issues of missing near-ground data and lack of internal structure in the real-world models generated solely based on this technique, this study explores two levels of research: the fusion of aerial and ground models, and the fusion of macro and micro models. Firstly, it reviews three main technologies: oblique photography, 3D laser scanning, and building information modeling. Then, it investigates the overall fusion processes and underlying technical principles (point cloud registration, data standards) at different levels. It compares and analyzes the advantages and limitations of relevant research and identifies their respective application scenarios. This study proposes two fusion technology frameworks to address the issues of real-world models under oblique photography, discusses the challenges faced by each framework, and provides prospects for their future development, aiming to serve as a reference for scholars in related fields.
Xun Xiaowei , Xu Xin , Pan Hongxia
2024, 47(10):48-55.
Abstract:To solve the problem that the traditional convolutional neural network (CNN) is not high in fault identification accuracy for spiral bevel gear box, an intelligent fault diagnosis method based on deep separation convolutional neural network (DCNN) and Bi-LSTM was proposed. Firstly, the original signal is denoised by wavelet threshold, and then decomposed by empirical mode decomposition (EMD) algorithm. Then, each component of the decomposed eigenmode function (IMF) is kurtosis calculated, and the IMF component with the highest kurtosis value is selected to construct a new vibration signal input model for training. After that, a large number of signal samples are obtained by overlapping the vibration signals, and the spatial feature information of these samples is adaptively extracted from the one-dimensional original vibration signals through the deep separation convolutional neural network. The extracted features are further input into the bidirectional long short-term memory network, and the forward and inverse time-domain vibration signals are extracted at the same time to better extract fault features. At the same time, the residual network is added to the deep separation convolution to reuse the data features, and the convolutional kernel is deeply separated to solve the network degradation problem of the deep model. Finally, the feature information is input into the trained DCNN-Bi-LSTM model to diagnose and identify the spiral bevel gear box fault. The results show that this method can accurately identify gearbox faults, and the highest diagnostic accuracy can reach 100%. Moreover, the proposed method has higher accuracy, stronger anti-noise ability, faster convergence rate and more stable diagnosis results than traditional convolutional neural networks.
Zhao Hailin , Liu Fuchao , Liu Ning , Zhao Hui , Wang Guiqi
2024, 47(10):56-61.
Abstract:In response to the significant challenges posed by multipath effects, signal attenuation, and frequent occurrences of gross errors and cycle slips in short-term occluded environments, which diminish the accuracy and robustness of vehicular satellite navigation systems, this paper proposes an integrated navigation method for vehicle GNSS/INS based on adaptive factor graph. Initially, a GNSS/INS factor graph model is established, utilizing INS as the primary navigation system, supplemented by GNSS factors for corrective adjustments based on GNSS measurement residuals. This design allows for adaptive adjustments to the weights of GNSS factors, effectively mitigating divergence errors caused by environmental changes and subsequently reducing the impact of excessive GNSS positioning errors on the combined navigation′s accuracy and robustness. The method was empirically validated using a racing car experiment, which demonstrated that the adaptive factor graph-based method significantly reduced the root mean square error and maximum error by 70.01% and 55.31%, respectively, compared to conventional factor graph-based methods. The results confirm that the proposed method enhances positioning accuracy and robustness.
Gao Pengfei , Li Tao , Xia Yongkang
2024, 47(10):62-69.
Abstract:Aiming at the problems of low efficiency and long time consumption in path planning of sparrow algorithm, an improved sparrow algorithm is proposed for path planning of inspection robots in distribution rooms. Firstly, using Logistic Tent chaotic mapping to optimize the quality of sparrow population and reduce subsequent blind searches. Secondly, a controllable adaptive random exploration discoverer update strategy was proposed to enhance the algorithm′s global search capability while further improving planning efficiency and shortening search time. Next, in order to avoid algorithm stagnation in the later stage, a spiral position update factor is introduced to enhance local development capabilities. Finally, combining cubic interpolation B-splines for smoothing processing makes the path more suitable for the distribution room environment. The experimental results show that the improved sparrow algorithm can efficiently complete the path planning task during inspections. Compared to the original algorithm, it is significantly optimized in terms of iteration efficiency, path search time, and other aspects.
2024, 47(10):70-77.
Abstract:The existence of eddy current loss leads to a reduction in the energy transfer efficiency and signal transmission quality of simultaneously wireless power and data transfer utilized for metal shaft monitoring. To address this issue, an analysis and derivation of eddy current loss in metal rotors were conducted. Subsequently, a coupling coil circuit model incorporating the equivalent impedance of eddy current loss was established. Building upon the S-S type signal injection SWPDT system, the energy transfer and data transmission characteristics were analyzed. Employing energy transfer efficiency and data transmission gain as optimization objectives, a multi-objective particle swarm optimization algorithm was utilized to optimize the critical parameters of the system. An experimental test platform was constructed based on the optimized parameters. The results demonstrated that the proposed optimization scheme achieves 46.7% energy transfer efficiency and a data transmission rate of 250 kbit/s in metal shaft environment, thus validating the correctness and feasibility of the proposed optimization approach.
Ni Hongqi , Li Xinyu , Dai Wenbo , Li Baoli
2024, 47(10):78-84.
Abstract:In order to achieve automatic detection of defects in metal hoses in industry, a deep learning based defect detection method is proposed. Firstly, a camera is used to capture images of defects in metal hoses, and the defect feature parts in the collected images are classified and calibrated. The surface defects of metal hoses can be divided into three types: broken wire, loose wire, and stacked wire, and corresponding self-made datasets are created; Secondly, the YOLOv5s network is improved by adding SimAM attention mechanism to the backbone network of YOLOv5s; Then use the EIoU loss function to replace the IoU loss function used by the initial network; Finally, the pyramid pooling layer in YOLOv5s was improved by replacing the SPPF module with the SimSPPF module. The improved algorithm was used to train the dataset of metal hose defects. Compared with the initial YOLOv5s network, the average accuracy mAP of the improved algorithm increased by 1.5%, and the missed and false detections of complex features and small targets were significantly improved.
Lu Siyuan , Shen Qinqin , Bao Yinxin , Gao Ruifeng , Shi Quan
2024, 47(10):85-92.
Abstract:Traffic flow prediction is a hot research area in intelligent transportation systems, and the fundamental challenge is to effectively model the complex spatial-temporal correlations in traffic data. To address the problem that most existing spatial-temporal Transformer models ignore the important effects of temporal trend and spatial heterogeneity when constructing spatial-temporal correlation matrices, a traffic flow prediction model based on Spatial-Temporal Aware Transformer (STAFormer) is proposed. First, an improved spatial-temporal aware self-attention mechanism is used to mine potential temporal trend and spatial heterogeneity features in traffic flow data, establishing an accurate spatial-temporal correlation matrix to obtain global spatial-temporal features. Then, the multi-range diffusion convolution is used to simulate the multi-order diffusion process of traffic flow in the road network to capture the local spatial features. Finally, the multivariate feature fusion module is used to adaptively fuse the captured spatial-temporal features and output the prediction results. Experiments are conducted on two real traffic datasets, i.e. PeMS04 and PeMS08, and the results show that, compared with the recently proposed Transformer-based models such as RPConvformer, ASTGNN, and PDFormer, the mean absolute errors of STAFormer are reduced by 8.0%, 6.5%, and 2.0%, respectively.
2024, 47(10):93-100.
Abstract:Aiming at the actual multi-robot formation control problems such as obstacle avoidance, external disturbance and hysteresis, a control method based on distributed reference correction is proposed. By using the artificial potential field method and the disturbance observer, the obstacle avoidance problem of mismatched uncertainty is solved. The unreachable reference scene is defined to describe the passive correction behavior of multiple robots when trying to avoid obstacles. A distributed reference correction algorithm is designed for each robot to reduce the adverse effects of passive correction behavior and ensure the boundedness of position tracking error of each robot. Considering the known actuator hysteresis effect, a Bouc-Wen hysteresis compensator is added to the current control law. The effectiveness of obstacle avoidance is verified by Lyapunov stability theory. Finally, numerical simulation and comparison are carried out based on the multi-robot system to prove the effectiveness of the proposed algorithm and controller. Finally, numerical simulation and comparison are carried out based on multi-robot system in MATLAB environment to prove the effectiveness of the proposed algorithm and controller. The experimental results show that the method can form a stable multi-robot formation control under practical problems such as external interference and hysteresis, and avoid obstacles on the path without collision. The proposed distributed reference correction algorithm weakens the passive correction behavior and improves the stability of the system.
2024, 47(10):101-107.
Abstract:Aiming at the problems of low diagnostic accuracy of SVM and easy to fall into local optimum of BWO algorithm in transformer fault diagnosis, a transformer fault diagnosis method based on EBWO-SVM is proposed. Firstly, the BWO algorithm is improved by introducing quasi-opposition-based learning strategy and cyclone foraging strategy, and then the EBWO algorithm and particle swarm optimization algorithm, grey wolf optimization algorithm, whale optimization algorithm, and beluga optimization algorithm are tested for optimality seeking on six test functions, which verifies the superiority of the EBWO algorithm. Secondly, the EBWO algorithm is used to optimise the kernel function parameters g and C in SVM so as to improve the classification ability of SVM. Finally other methods are proposed to compare with the EBWO-SVM model. The results indicate that the constructed EBWO-SVM transformer fault diagnosis model improves the comprehensive diagnostic accuracy by 7.7%, 9.7%, 11.6%, and 15.4% compared with BWO-SVM, WOA-SVM, GWO-SVM, and PSO-SVM, respectively, and is more stable, which verifies the feasibility and effectiveness of the EBWO-SVM model.
Cheng Jie , Sha Yuebing , Zhou Yiming , Cheng Yinbao
2024, 47(10):108-114.
Abstract:Precision instruments are tending towards intelligence and diversified functions, making the evaluation of measurement uncertainty in precision instruments more complex. Taking roundness measurement in CMM as an example, a task-oriented uncertainty evaluation method is used to quickly and reliably evaluate the uncertainty of CMM measurement tasks. Based on the actual situation of error propagation and combined with the Monte Carlo method of computer simulation, a method for algebraic sum synthesis of uncertainty under multiple states of error distribution is proposed. Aiming at the problem of overestimation in the initial evaluation of measurement uncertainty, an optimized evaluation scheme is proposed. Provide an example of uncertainty evaluation and optimization for roundness measurement tasks to verify the feasibility and effectiveness of the evaluation model and optimization methods. Systematically solving the uncertainty evaluation problem of CMM task oriented has important reference significance for solving the uncertainty evaluation of other high-precision instrument measurement results and improving the practical use value of the instrument.
Mao Lin , Ren Chunhe , Yang Dawei
2024, 47(10):115-125.
Abstract:Aiming at the problem that the existing human pose estimation algorithm has insufficient feature extraction of the backbone network, which leads to the loss of key point feature information, a human pose estimation network model (GLF-Net) combined with global-local feature fusion module is proposed. In order to obtain high-quality feature maps in the feature extraction stage, the algorithm improves the backbone network ResNet-50 from the global and local features, and designs a global polarization self-attention module and a local depth separable convolution module respectively. At the same time, a parallel structure is used to embed the module that combines global position information and local semantic information features into the Bottleneck layer of the backbone network, which can not only enhance the feature extraction ability of the original backbone network, but also provide effective global and local feature input for the subsequent Transformer network, thereby improving the performance of pose key point detection. The model test is carried out on the public human pose estimation dataset COCO 2017 and MPII dataset respectively. Compared with the benchmark algorithm (Poseur), the average accuracy of the pose key points is increased by 2.1%, the average recall rate is increased by 1.5%, and the proportion of correctly estimated key points (PCKh@0.5) is up to 90.6. The experimental results show that the proposed algorithm is superior to the existing similar methods in the accuracy of pose estimation, and can significantly improve the positioning accuracy of human pose key points.
Lin Lipeng , Yang Zhaoyang , Wu Mingcheng , Wang Renping , Yin Yadong
2024, 47(10):126-133.
Abstract:In order to solve the problem of high hardware resources consumption in the CLAHE algorithm, two improvements are made to the algorithm from the perspective of hardware implementation. For the cropping thresholds, a method for determining the universal clipping threshold is proposed.The Figure Of Merits(FOM) is constructed based on Information Entropy and Structural Similarity, and the clipping threshold in hardware implementation is set using the best FOM as the evaluation criterion to balance the contrast and distortion degree of the image enhancement and avoid consuming hardware resources to perform extensive calculations on the image data itself. For the redistribution of pixels exceeding the threshold, an improved superthreshold pixel redistribution method is proposed, which only distributes pixels to grayscale levels that do not exceed the threshold and stops redistribution if they exceed the threshold again. This method reduce the distortion while avoiding hardware costs caused by repeated pixel distribution. On the basis of the improved CLAHE algorithm, the low illumination image enhancement system based on FPGA is completed. The experimental results show that image enhancement processing based on the universal cropping threshold can generally achieve higher FOM, thus having better overall image enhancement effect. Compared with conventional methods, the improved pixel redistribution method can increase the average SSIM of the image by 8.88% at the cost of an average IE loss of 3.28%. The low light image enhancement system can achieve image acquisition and processing of 640×480@60 fps. This design can provide a new reference for the hardware implementation of image enhancement algorithm.
Li Bo , Li Zhikang , Zhou Yubin
2024, 47(10):134-140.
Abstract:In order to solve the problem that the existing SAR target detection algorithm only uses the underlying features of the image for detection, and the detection rate of small-scale ship targets is low, an object detection algorithm combining feature fusion and attention mechanism was proposed. For SAR ship-target detection, on the basis of the original backbone network SSD target detection algorithm, the attention mechanism module is introduced, the feature maps at different levels are fused with features, the images containing small-scale targets are oversampled, and the data augmentation is achieved by copying and pasting small targets multiple times. Through a large number of training and testing of SAR ship image datasets, the results show that the proposed algorithm can effectively improve the comprehensive detection performance of ship targets, and the mean average precision can reach 94.16% on the public SAR ship target detection dataset.
Zhang Yu , Wang Yanji , Ma Hui , Yan Kai , Li Dazhou
2024, 47(10):141-150.
Abstract:In the fields of UAV reconnaissance, security monitoring, and autonomous driving, target detection technology faces significant challenges. Targets in images often exhibit multi-scale attributes, making detection of small-sized targets particularly difficult, and targets are prone to various degrees of occlusion. To address these pressing issues, this paper proposes an innovative dynamic multi-scale target detection model: YOLO-DDE. Firstly, novel CEMA and CED convolutional modules are introduced to enhance the backbone network′s ability to handle multi-scale information and extract fine features, thus achieving more precise recognition in complex scenes. Additionally, the FPAN network structure is innovatively restructured into the DFPN structure, which employs longitudinal cross-scale fusion technology to significantly improve the model′s scale feature fusion effect.Finally, a dynamic detection head is introduced, proposing the DD-Head structure, which strengthens the model′s ability to handle downstream tasks. In summary, the proposed YOLO-DDE model, with its dynamic multi-scale structure, provides new possibilities for improving target detection technology performance.Experiments on the PASCAL VOC dataset were conducted to validate the proposed model. Compared to the current state-of-the-art model YOLOv8, the YOLO-DDE model achieves a 1.8% and 3.2% improvement in evaluation metrics map50 and map50.95, respectively. Furthermore, generalization experiments on the VisDrone, HIT-UAV, and FAIR1M2.0 datasets validate the model′s strong generalization ability.
Li Bing , Du Xiying , Wang Yuying , Zhai Yongjie
2024, 47(10):151-159.
Abstract:The substation equipment is an important component of the power transmission and transformation process of the power grid. To ensure the normal operation of the power grid, it is necessary to diagnose faults in substation equipment, and accurate segmentation of substation equipment in infrared images is a key step in fault diagnosis. In response to the low segmentation accuracy and missed segmentation issues faced when segmenting substation equipment in complex scenes of infrared images, an instance segmentation algorithm for substation equipment in infrared images based on improved YOLOv8n is proposed. Firstly, a context-guided feature-enhanced down-sampling block is designed to replace the down-sampling convolutional layer in YOLOv8n, fully utilizing contextual and global information to enhance the model′s understanding of complex scenes. Then, the C2f module is introduced to reconstruct the deformable convolution in the Backbone, enhancing the extraction capability of irregular equipment features. Finally, the loss function is optimized using Wise-IOUv2 to improve the model′s generalization and classification ability. Using the infrared image dataset of substation equipment to experimentally verify the model, the experimental results show that compared to the YOLOv8n baseline model, the proposed method in this paper has increased mAP50 and mAP50:95 by 4.2% and 3.5% respectively. The proposed method can better solve the problem of missed segmentation of equipment in complex scenes and effectively improve the accuracy of substation equipment instance segmentation.
Song Yijiao , Kong Huihua , Li Jian , Qi Ziwen , Zhang Ran
2024, 47(10):160-167.
Abstract:Overpressure tomography is a typical incomplete data reconstruction problem, which uses the shock wave signal collected by the sensor to invert the overpressure distribution in the test area. In order to improve the solution accuracy, a shock wave overpressure tomography reconstruction method based on Gauss-Newton iterative combined superlaplace regularization is proposed in this paper. Because the collected shock wave signal is usually aliased with the interference signal, which will affect the measurement accuracy of the overpressure value, this paper firstly adopts the improved wavelet threshold algorithm to denoise the shock wave signal. Secondly, the image edges and the two-dimensional chromatographic model are constrained by the superlaplace prior. Then Gauss-Newton iterative algorithm and alternating direction multiplier algorithm are used to solve the problem of large ill-conditioned sparse matrix. The experimental results show that compared with the traditional total variational regularization and total generalized variational regularization, the reconstruction accuracy of the proposed method can be maintained at about 15%, which has certain application value in practical scenarios.
Lin Enhui , Wang Fan , Tan Xiaoling
2024, 47(10):168-174.
Abstract:In the field of facial expression recognition, low parameters and high accuracy are difficult to complete together, A facial expression recognition method based on ShuffleNetV2 network combined with attention mechanism is proposed in this study. Based on ShuffleNetV2 architecture, this method further improves the feature capture and classification capability of the model by fine-tuning the model and replacing the Relu activation function with the PRelu activation function. In addition, this paper introduces an ultra-lightweight dual attention module LDAM, which combines DCAM attention mechanism and spatial attention mechanism, and integrates it into the optimized ShuffleNetV2 model by shortcut connection technology, so as to enhance the model′s recognition ability and classification effect of detailed features. Experimental results on FER2013 and CK+, two widely recognized facial expression recognition datasets, show that the proposed method achieves recognition accuracy of 69.12% and 94.77%, respectively, while maintaining a model parameter count as low as 1.25. This result is not only demonstrated in the possibility of maintaining the lightweight model while improving the recognition performance, but also verifies the efficiency and practicability of the proposed method through experiments.
Wang Xiaxia , Zhang Xuan , Wu Xinglong , Huang Qing , Xu Guoping
2024, 47(10):175-183.
Abstract:In the field of medical image segmentation, noise interference from different devices or parameters negatively affects the generalization of the model. To solve the problem of segmentation model performance degradation caused by different noise sources, a two-stage efficient segmentation model NANet with adaptive noise pollution is proposed.The model mainly consists of two parts: denoising and segmentation. The denoising part uses an unsupervised U-shaped autoencoder structure combined with a frequency-domain learnable denoising module to mitigate the noise-induced target-domain differences. The segmentation part builds modules based on the DeepLabv3+architecture, focusing on improving the segmentation accuracy and generalization ability of targets in noise-polluted ultrasound images. Experiments were conducted to test the segmentation performance of the model on four different ultrasound datasets contaminated with uniform, Gaussian and pretzel noise. The experimental results show that the Dice coefficients of the proposed NANet for segmentation on the original image of the brachial plexus neural ultrasound dataset, the image data contaminated by uniform, Gaussian, and pretzel noises are 79.71%, 78.51%, 79.75%, and 79.1%, respectively. Comparison experiments show that the segmentation performance of NANet on ultrasound images with different noise types is significantly better than that of traditional segmentation methods, in which the accuracy is improved by more than 10% compared to U-Net, DeepLabv3+, and AttUNet methods on both Gaussian and pretzel noise images. The results validate the robustness and generalization ability of the proposed NANet in different noise environments.
2024, 47(10):184-190.
Abstract:Existing DCNN generate a large amount of inter-layer feature data during inference. To maintain real-time processing on embedded systems, a significant amount of onchip storage is required to cache inter-layer feature maps. This paper proposes an inter-layer feature compression technique to significantly reduce off-chip memory access bandwidth. Additionally, a generic convolution computation scheme tailored for BRAM in FPGA is proposed, with optimizations made at the circuit level to reduce memory accesses and improve DSP computational efficiency, thereby greatly enhancing computation speed. Compared to running MobileNetV2 on a CPU, the proposed DCNN accelerator in this paper achieves a performance improvement of 6.3 times; compared to other DCNN accelerators of the same type, the proposed DCNN accelerator in this paper achieves DSP performance efficiency improvements of 17% and 156%, respectively.
Lu Wenxiang , He Xiaohai , Yun Xutuo , Teng Qizhi , Lyu Zhaoyang
2024, 47(10):191-198.
Abstract:The traditional registration algorithm has low registration accuracy and slow registration speed on high-resolution rock microscopic images. In addition, in the process of panoramic stitching of microscopic rock slices, due to the lack of effective content in single-view images, effective feature points cannot be extracted, or there are fewer feature points extracted by a single texture, resulting in panoramic image stitching failure. Aiming at the above problems, a BRISK image stitching algorithm based on an improved phase correlation method is proposed. By extracting the similar regions between the images to be registered, the BRISK image registration is performed on the similar regions, and the best matching model obtained by the motion law of the stage is used to register the image without feature points. Finally, the panoramic mosaic of rock slice images is completed. The experimental results demonstrate that the algorithm proposed in this paper achieves a registration accuracy of 87.5% compared to traditional algorithms, with a registration time of 2.662 seconds per pair. It exhibits advantages in terms of both fast registration speed and high accuracy. Moreover, this paper presents a method for panoramic stitching of rock thin sections, which offers practical value for real-time stitching applications.
Editor in chief:Prof. Sun Shenghe
Inauguration:1980
ISSN:1002-7300
CN:11-2175/TN
Domestic postal code:2-369